A digital twin framework for machine learning optimization of aerial fire fighting and pilot safety
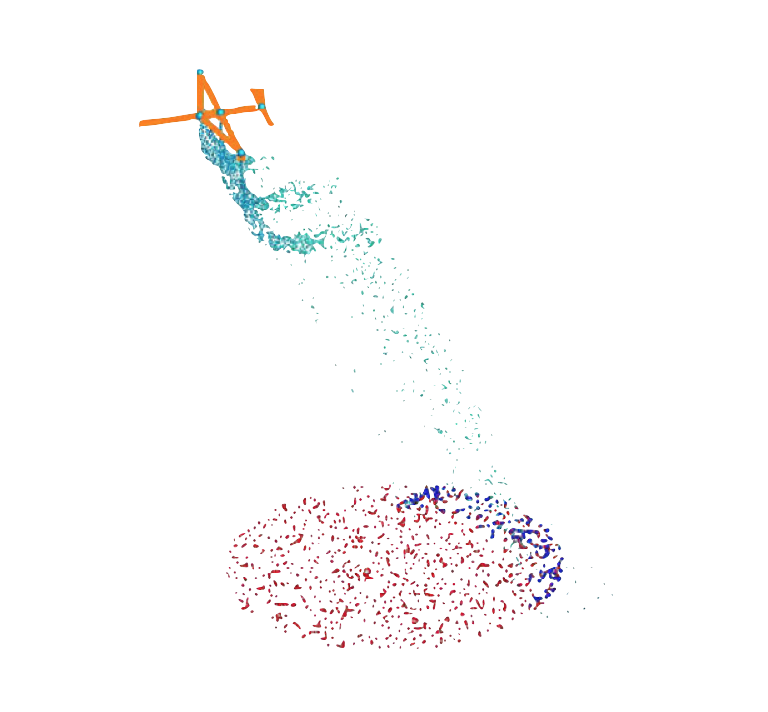
Abstract
The objective of this work is to model and simulate aerial drops of fire retardants in dangerous fire environments. Specifically, the work develops a computational framework for a model problem combining:
1. A meshless discrete element component that tracks the trajectory of released airborne materials from a controlled aircraft, ranging from retardant powders to encapsulated packets, subjected to prevailing wind velocities and fire-driven updrafts.
2. A Machine Learning Algorithm (MLA) to rapidly ascertain the optimal aircraft (unmanned or manned) dynamics to maximize the fire-retardant release effectiveness (released material usage and target impact).
The framework is designed to enable Digital Twin type technologies, i.e. digital replicas that run in real time with the physical system. However, it is also designed to run at much faster rates, in order to enable MLA’s to optimize the planning, by running quickly on laptops and mobile systems. The overall guiding motivation is to provide a useful tool to enable rapid flight-path planning for aerial first-responders in real-time and to train pilots. Numerical examples are provided to illustrate the process.
Relevant Publications
181. Zohdi, T.I. (2020) A digital twin framework for machine learning optimization of aerial fire fighting and pilot safety, Computer Methods in Applied Mechanics and Engineering. https://doi.org/10.1016/j.cma.2020.113446